Architectural Design
There are two major components that the Ollama Operator will create for:
- Model Inferencing Server: The model inferencing server is a gRPC server that runs the model and serves the model's API. It is created as a
Deployment
in the Kubernetes cluster. - Model Image Storage: We need a service to store the downloaded models and re-use them instead of downloading their own each time requested, a
StatefulSet
along with aPersistentVolumeClaim
will be created. And the image will be stored in a dynamic provisionedPersistentVolume
.
Why do we need an extra Model Image Storage?
The image that created by Modelfile
of Ollama is a valid OCI format image, however, due to the incompatible contentType
value, and the overall structure of the Modelfile
image to the general container image, it's not possible to run the model directly with the general container runtime. Therefore a standalone service/deployment of Model Image Storage is required to be persisted on the Kubernetes cluster in order to hold and cache the previously downloaded model image.
Although it's a standalone service, we are not deploying large registry server like Docker Registry or Harbor. What Ollama Operator will do, is simply start a server with the ollama serve
built-in command. At the time where each model inference service got created, a image pull request will be sent directly to this service.
The detailed resources it creates, and the relationships between them are shown in the following diagram:
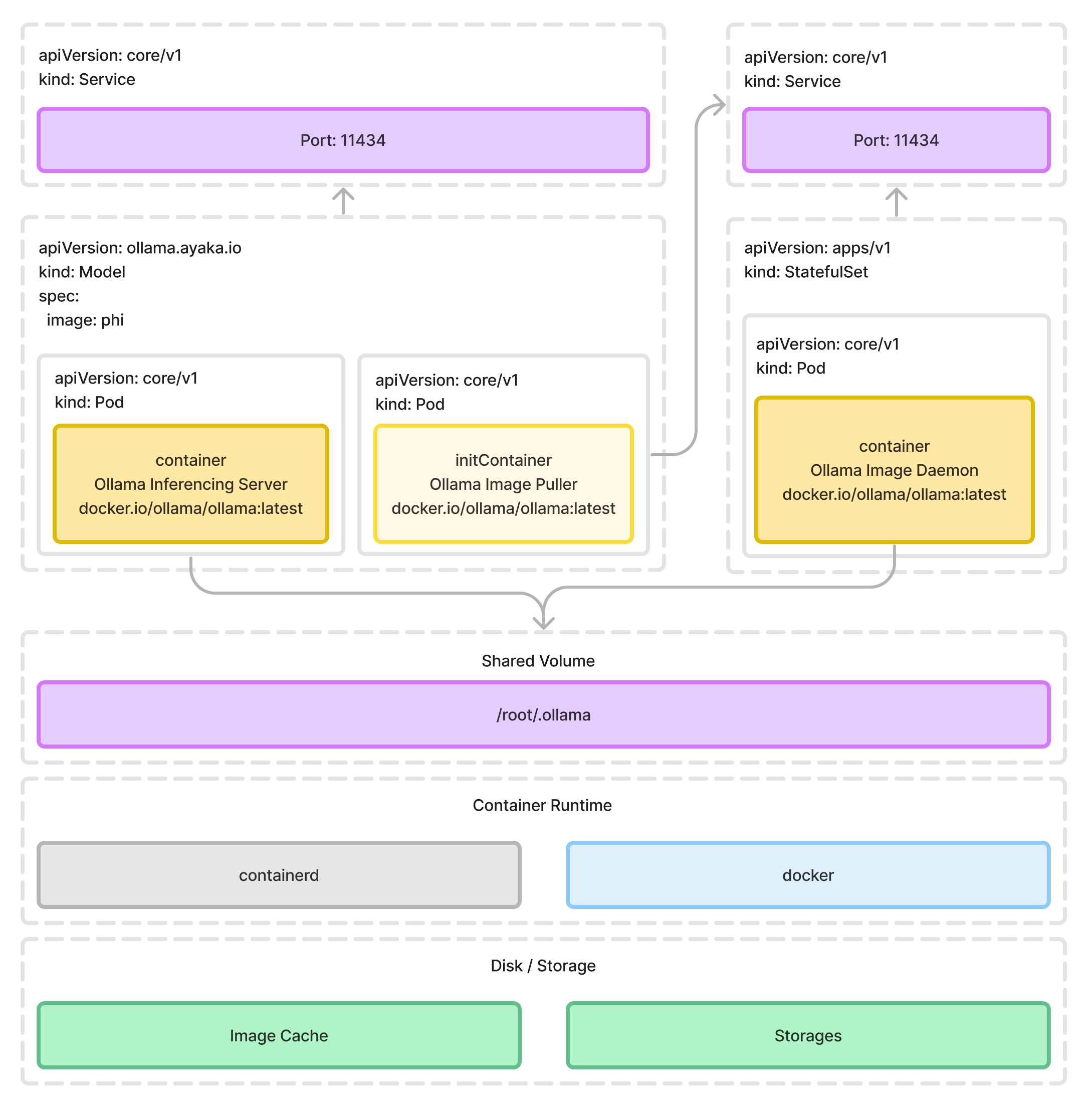